Amazon
- Suphanet Kotchum
- May 6, 2021
- 9 min read
Among many crucial problems that the COVID-19 pandemic has shed some light on is how companies treat their employees and workers, especially during this difficult time. With company after company laying off their employees, Amazon has been one of the few that did the opposite. News of Amazon going on a hiring spree for corporate employees and warehouse workers continuously made the headlines. However, alongside these headlines are those on how the company has been mistreating their warehouse workers.
Dated back to even before the times of COVID, big corporations like Amazon or Apple have always been in the spotlight for workplace satisfaction. These companies typically promote themselves as employers who genuinely care for their workers and employees and offer a favorable and supportive workplace environment. Despite that, workers exposing these employers for exploitation and mistreatment of their employees through social media platforms and news outlets. For this project, the focus is to examine Amazon workers’ workplace satisfaction and reference any reputation changes due to the mistreatment headlines during the pandemic.
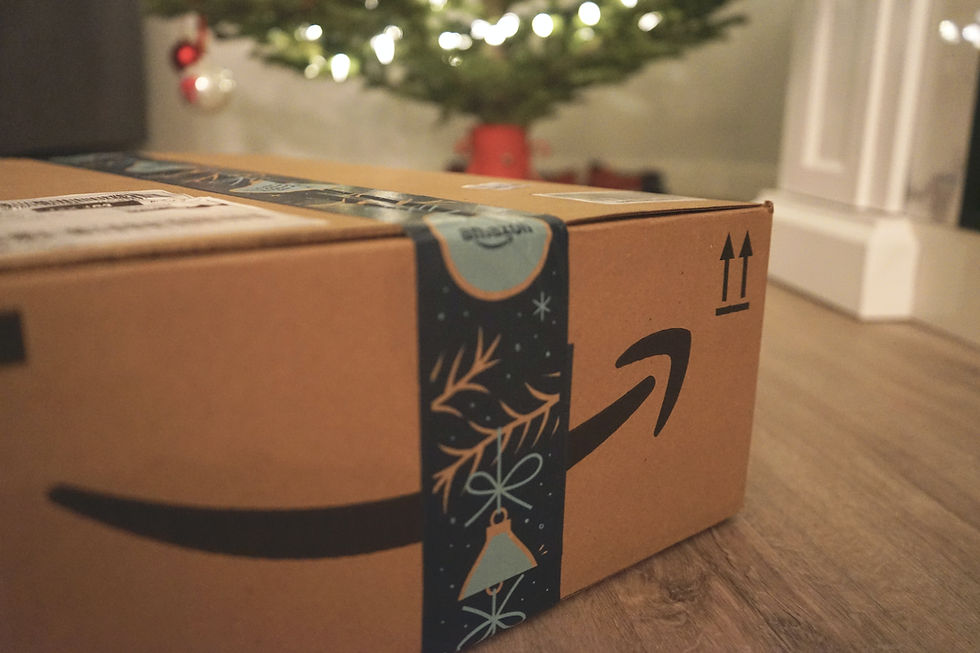
Employees Mistreatment and Muted Voices in the Culturally Diverse Workplace
With the innovations of technology and the internet, both employees and employers learn that one of the crucial critical points to company culture is diversity and equality. Although many companies promote themselves as such, mistreatment still exists within today's workplace culture. This study examined the perspectives of employees on their own experience with workplace mistreatment. While some employees can freely speak up, some others are muted due to their lower positions within the company or their cultural backgrounds.
Workplace Mistreatment Climate and Potential Employee and Organizational Outcomes: A Meta-Analytic Review From the Target’s Perspective
This study dived deeper into understanding the context to workplace mistreatment climate, or MC as referred to in the study. Many employers today have brought up the issue of mistreatment and workplace inequality to the spotlight. They have actively looked for ways to implement policies and procedures to prevent MC from happening within the company. Despite this, mistreatment can come in the form of interpersonal, “…which are often subject to individual interpretations and the influence of dynamic relationship quality.” (Yang 2014) Due to this, MC is often not consistently examined within an organization and leaves space for mistreatment. Understanding the full scope and context of MC, or mistreatment climate, can help an organization know what they need to do to create a better work environment.
Research Questions
Research Question 1:
Are Amazon warehouse workers satisfied with their work environment?
Null hypothesis: Amazon warehouse workers are satisfied with their work environment.
Alternative hypothesis: Amazon warehouse workers are not satisfied with their work environment.
Research Question 2:
How has Amazon’s reputation changed recently?
Null hypothesis: Amazon’s reputation did not change.
Alternative hypothesis: Amazon’s reputation has gotten worse recently.
Data Description and Justification
Employment Reports Published by Amazon
The employment report data, published by Amazon, consists of three scraped web pages. This data was included in the analyzes, to understand how Amazon portrays itself. It was included to make the report more well-rounded and to be able to analyze whether there is a difference between how Amazon views itself compared to how employees, the media, and the public view them.
Tweets
Two different tweets datasets were pulled from the Twitter API into data frames using the “rtweets” package from R. One of them focused on the keyword “Amazon employees”, while the other focused on “Amazon workers”. Each dataset contains a total of one week’s worth of tweets. Considering that it is not feasible for us to conduct interviews with Amazon employees and warehouse workers to collect data, the tweets datasets will be used as one of the three first-hand sources as to whether Amazon employees and workers are satisfied with their current work conditions.
The raw datasets contain Twitter users’ publicized account information, such as their screen_name (username), name (display name), location, description, followers_count, et cetera. They also include information on the tweets, such as status_url, media_url, retweet_favorite_count, and many more. The screen_name, text, and lang are selected to subset into new datasets for cleaning and analyzing. Screen_name consists of usernames for each of the Twitter users who post the tweets. Text contains all the tweets that were pulled from the Twitter API. Lastly, lang indicates the language that the tweets were in. Including the language information in the new dataset will help with understanding and cleaning the data later on. The text column will be renamed as “tweet”, and the lang column will also be renamed as “language” for better clarification.
The eight raw Reddit datasets were randomly picked based on their relevance to Amazon and its employees or warehouse workers. These datasets were pulled from Reddit API through an R client package called “RedditExtractoR”. Each dataset contains information about the Reddit users and the posts, including post_date, comm_date, subreddit, user, title, et cetera. Since Twitter API only allows easy free access to up to one week of data, Reddit datasets will also be included as a second source of first-hand account to whether employees are satisfied with their current work environment.
All eight datasets were then combined and subsetted into a new dataset that only contains user and comment for further data cleaning and analysis. Including the user component will help with the analyzing process later on, as it can be grouped to understand the sentiments of users’ word choices. In the clean Reddit dataset, the comment column will be renamed as “clean.reddit” for better clarification.
Glassdoor
The Glassdoor dataset was picked on the article and reviews about Amazon Reviews by their full-time and part-time employees. The Glassdoor API contains information of its employees whether how long they have been at the company and in which position. This dataset was pulled from the Glassdoor API through an R client package called “rvest”.
Each data set contains information about the list of Glassdoor users, the pro reviews and the con review about how employees feel about Amazon. The pro and con reviews are combined into a new column in the dataset as “reviews” for further text mining analysis.
News
The news dataset contains 80 scraped news articles from the New York Times at 4/10/2021 related to Amazon. The urls and time are extracted using the nyt_search API with the search term Amazon.com. Then each of the url will be sent to the web scraping API from rvest package to extract the articles. After concatenating the sentences, each article will be compressed into a single string and transferred into the data file for further analysis.
Justification for Choice of Analytical Technique(s)
Text Mining
Text Mining analysis is used in this project to discover new information for the research questions so that the opinions on what Amazon workers/employees have on Amazon are received. The sentiment analysis is incorporated to understand the polarity, negative, positive, or neutral context and the emotions most people feel toward Amazon. Then, the next step is also specifically looked into negative words to spot bad/harmful words.
- Sentiment analysis:
● Bing: for positive and negative words
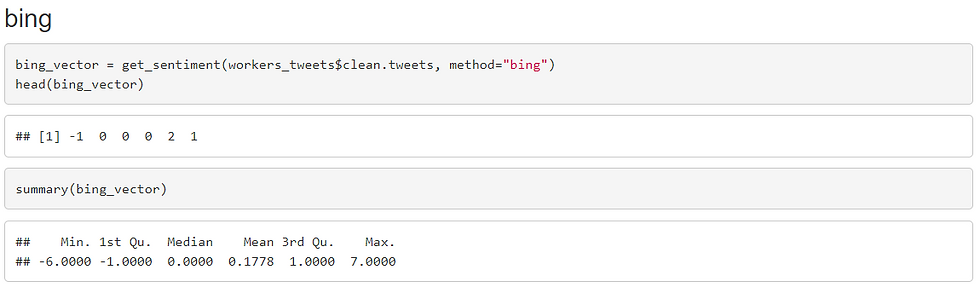
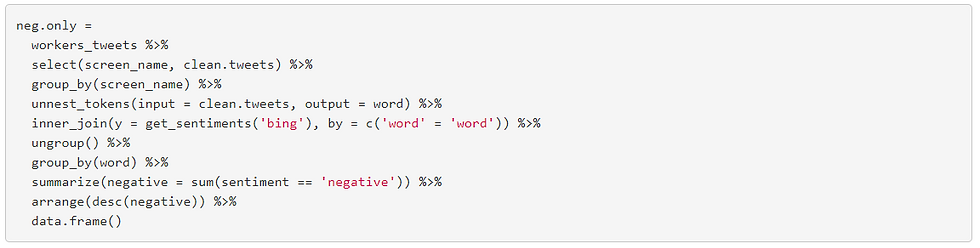
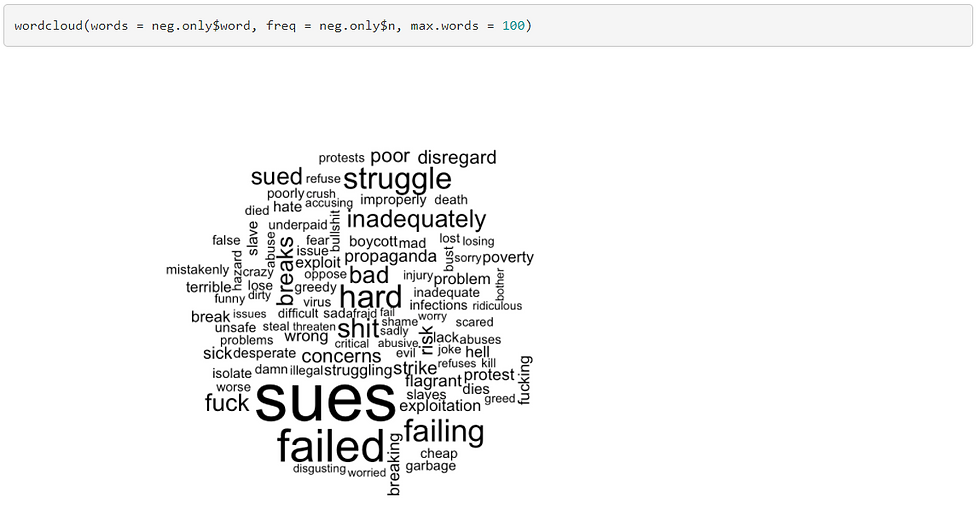
● NRC: for emotion words in reviews

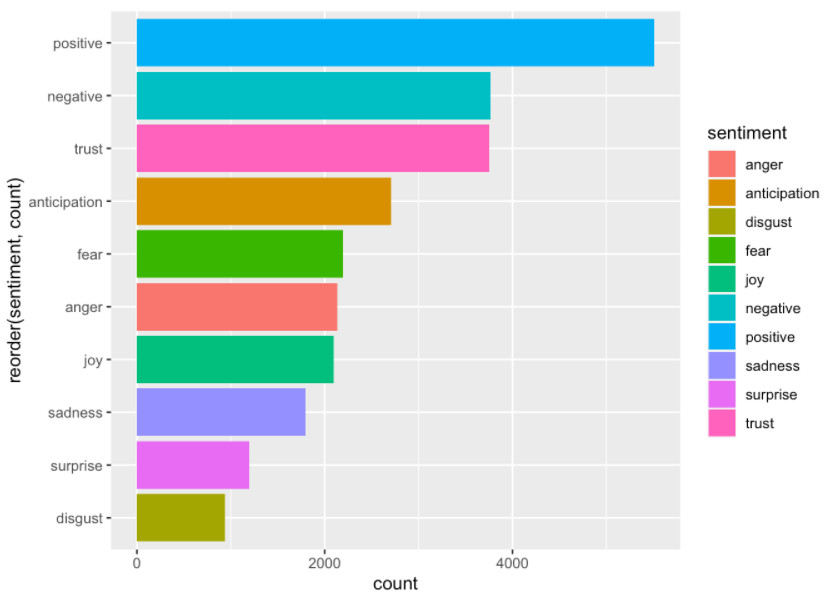
● Afinn Lexicon Method: for positive and negative percentage
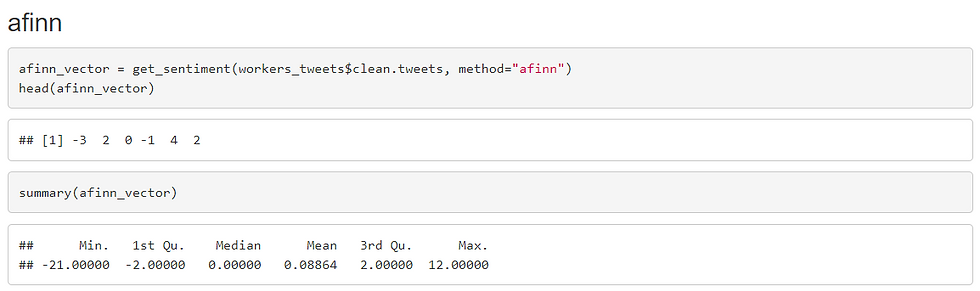
- Topic model: to analyze a group of words from the collection of documents that best represents the information on the collection. relationships between a set of documents and the terms they contain by producing a set of concepts related to the documents and terms.



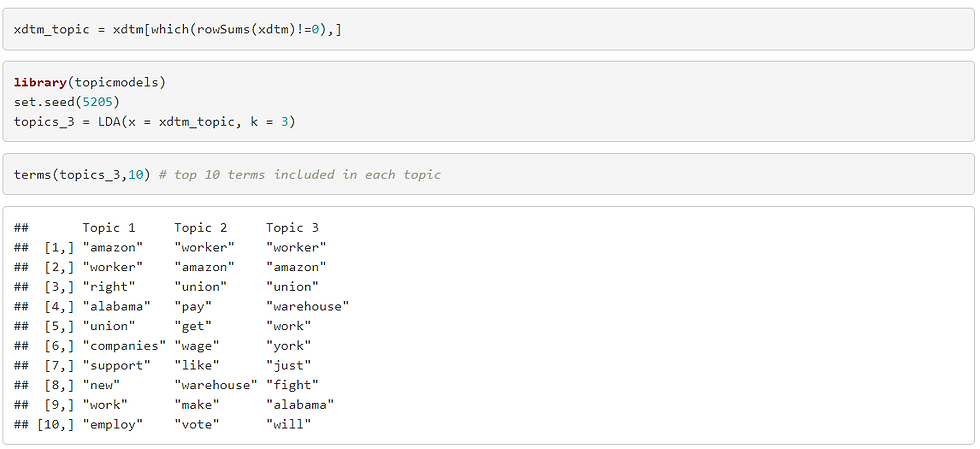

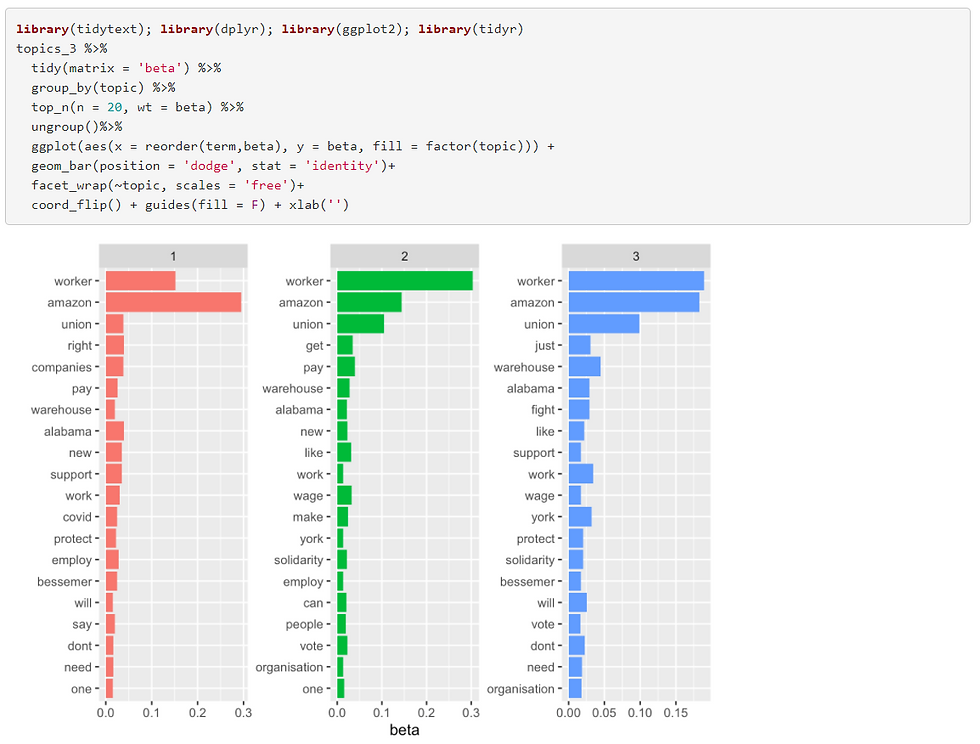
Time Series
Time series analysis is employed in the process to extract meaningful statistics and other characteristics of the Amazon’s reputation over the periods of time.
- ACF and PACF were used to understand the correlation of series with the lagged values.
- ARIMA was used to measure the Amazon’s reputation over a period of time






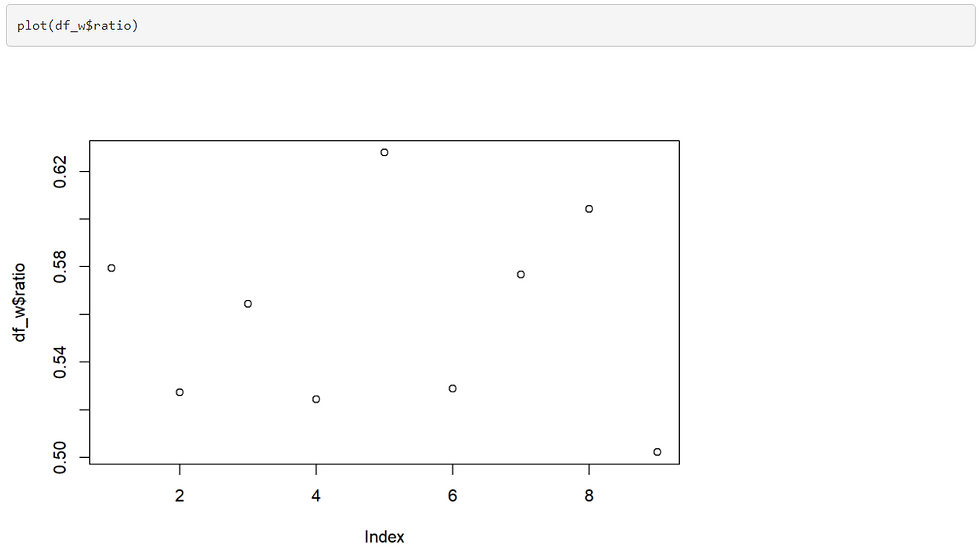
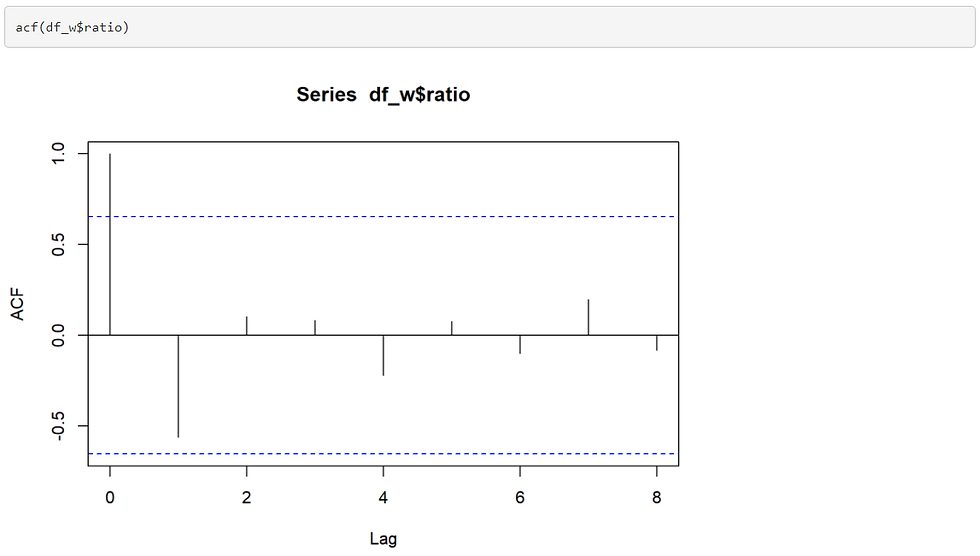

Results
Research Question 1:
The tweets dataset, Reddit dataset along with the Glassdoor dataset were used to conduct our analysis for research question 1. The initial results obtained from the text mining analysis shows that despite some recent bad headlines on Amazon’s mistreatments of their workers, the users of these platforms still seem to choose very positive wording choices when talking about the company. There are a larger number of choices in positive wording along with words that portray positive emotions, compared to the negative ones.
Even so, taking into account that the words might be wrongly interpreted by the system due to being taken out of context, the analysis went deeper by looking at the top 100 negative words that people used to talk about Amazon. While many of them are the typical curse words that can expect from internet (social media) users, there are also some heavy use of strong, negative words that point to the possibility that mistreatment problems might really exist at Amazon.
The text modeling was used for Reddit and tweets datasets, and their results also point in a similar direction that the majority users from these two platforms seem to be talking about standing in solidarity with unionizing Amazon’s warehouse workers. Lastly, the calculation of the average in word choice positive/negative ratings was incorporated to get an idea of the overall sentiment in these datasets. Both the “bing” and “afinn” methods produced similar results that on average, the word choice is quite evenly distributed between positive and negative.
Research Question 2:
According to the time series analysis on the average weekly sentiment value of the New York Times news articles generated using the bing sentiment lexicon from the tidyverse library, there is no change in sentiment value about Amazon.com recently. Then, Amazon's reputation in one of the most influential media has not been drastically changed recently.
The conclusion is drawn using 3 methods. By examining the scatter plot, the average sentiment for each week is randomly scattered across 0.55 and a clear linear relationship between the average sentiment value and time cannot be seen. Then, the next step was examining the ACF and PACF plots of the average sentiment value by week using acf and pacf functions, and there is no significant ACF or PACF correlation at any lag except for ACF at lag 0, which indicates that the change of average semantic value is behaving like a white noise process.
Lastly, the auto arima function was deployed to examine the time series, but the returned resulting model is an ARIMA(0,0,0) model with the mean coefficient of 0.5595, which also indicates that the change in average sentiment value over time is behaving like a white noise process. Therefore, we may say that there is no change in average sentiment value about Amazon.com over time recently according to the news articles and the overall average semantic value is the best prediction for Amazon.com’s reputation.
Limitations
The results found in this study might not accurately represent the satisfaction rate of Amazon workers with their workplace environment. There are two major limitations to our study, including the lack of first-hand account data and the inability to conduct sarcasm detection for our text mining process.
Lack of First-hand Account Data
The best way to measure workers’ satisfaction is to get direct feedback from the people who are actually working at Amazon. The result would have been more accurate if interviews or surveys were able to be conducted. However, due to the limitations of the time on the project, conducting interviews was not feasible.
For the study, the analysis includes a week’s worth of tweets, eight different related Reddit posts along posts scraped from Glassdoor as the first-hand account to determine workplace satisfaction. Taking into account that not users who posted or commented on Twitter and Reddit might be current or former employees, these datasets alone might only reflect the satisfaction of a small population of Amazon workers.
Sarcasm
From the analysis, there seems to be more of a positive trend in the word choices when people talk about Amazon per the datasets we have gathered on Twitter, Reddit, and Glassdoor. However, it is important to note that this result might be positively skewed due to the inability of detecting sarcasm in text mining process.
Users of both the Twitter and Reddit platforms tend to be sarcastic about what they say. This sarcasm can be portrayed in many different ways. By being able to only dissect and analyze word by word, the words users chose might be wrongly interpreted by the system and categorized into the positive groups, while in full context, they do not reflect positive emotions.
Conclusion
Due to the limitations in the study, a definite answer to Amazon’s mistreatment of workers cannot be clearly identified. However, based on the results in the text mining analysis, there were heavy usage of strong, negative words that indicate there is an issue within Amazon’s work environment and the company’s culture. One trend that can be seen throughout the analysis is that people do seem to be pushing for unionizing the warehouse workers. This might also be an indication that workers are not as satisfied with the company as it might seem through the positive word choice that we have obtained in the initial results.
The results for the second research question also point in a positive direction for Amazon, noting that there was no change in Amazon’s positive reputation in recent months, despite the workers' mistreatment headlines. This might be because Amazon’s business has been booming during the pandemic, as demands for online shopping goes up. However, considering the text mining analysis, it is important that Amazon also recognize that there might be a problem brewing below the surface. With society now more conscious of human rights and workplace culture, these problems could potentially result in loss of business for Amazon due to consumers not wanting to support a business that exploits its workers.
Recommendations
As this study was not without its limitation, due to not having access to broader and more long-term data, this report shall be used as a starting point only. The suggestion is that this matter be investigated more deeply and on a continuous basis. There might not be a serious issue of mistreatment now, however it left unchecked there is the possibility that one develops. It is recommended that the company culture across the board be looked at, as there might be a difference in how warehouse workers are treated compared to corporate employees, which can lead to resentment between different employees. Which in turn will poison company culture. It is proposed that a commission, that contains Amazon employees from across the company, is created to investigate this matter and proposes and enact reforms as it sees fit.
References
Meares, Mary M, et al. “Employees Mistreatment and Muted Voices in the Culturally Diverse
Workplace.” Journal of Applied Communication Research, vol. 32, no. 1, Feb. 2004.
Yang, Liu-Qin, et al. “ Workplace Mistreatment Climate and Potential Employee and
Organizational Outcomes: A Meta-Analytic Review From the Target’s Perspective.”
Journal of Occupational Health Psychology, June 2014.
Reddit posts
reddit1
reddit2
reddit3
reddit4
reddit5
reddit6
reddit7
reddit8
Amazon:
R Documentation:
“Rtweet” package
“RedditExtractoR” package
“Rvest” package
New York Times Search API
Comments